9 Strategies for Quickly Learning New Data Analysis Tools
Navigating the myriad of data analysis tools can be a daunting task, but it doesn't have to be. This article demystifies the process, offering practical strategies derived from the wisdom of seasoned industry experts. Embark on a journey to swiftly master new data analysis tools and elevate your analytical prowess.
- Create Simple Problems for Hands-On Learning
- Dive into Targeted Pet Projects
- Integrate Tools into Existing Projects
- Adopt Structured Yet Flexible Approach
- Start with a Familiar Dataset
- Blend Theory with Practical Application
- Get a Grip on Main Ideas
- Identify Specific Use Cases
- Combine Hands-On Practice with Structured Learning
Create Simple Problems for Hands-On Learning
When learning a new data analysis tool or technique, I would create a simple, easily visualizable problem for myself and try to solve it. Instead of just following courses and tutorials, I would learn by doing, experimenting with real or synthetic data to understand how the tool works in practice. I also like to use a pencil and notebook to sketch the problem and data structure, which helps me see relationships more clearly. This hands-on approach allows me to grasp key concepts faster while troubleshooting along the way. Additionally, I would refer to official documentation, online resources, and community discussions to deepen my understanding and refine my solutions.
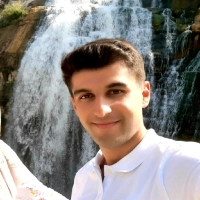
Dive into Targeted Pet Projects
I've found the most effective way to master a new data analysis tool or technique is to dive straight into a targeted pet project. Rather than getting bogged down in documentation upfront, I select a small, focused project that aligns with our business needs and utilizes the tool's core capabilities. For instance, when we were exploring Apache Superset for visualization, I started by building a simple dashboard tracking our quote-to-bind ratios. This was an easy calculation, but required getting to know the tool and seeing its capabilities while delivering some immediate business value. This approach naturally leads one to the relevant documentation exactly when needed. I've found that learning through practical application helps the concepts stick because the brain can immediately connect them to real-world scenarios. Instead of abstract studying, solving concrete problems makes the learning process both more efficient and enjoyable.
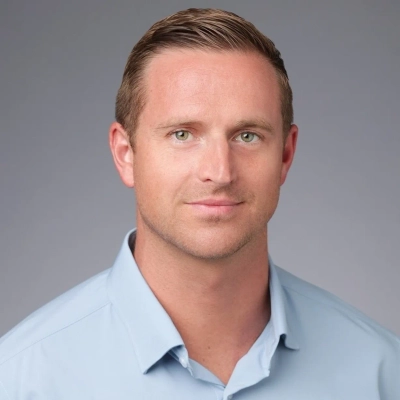
Integrate Tools into Existing Projects
When I need to learn a new data analysis tool or technique, I rely on integrating it into existing projects for immediate application. For example, when developing HUXLEY, our AI business advisor, I introduced AI-driven analytics tools like Qlik Sense into our operations to unify and visualize cross-platform data. This hands-on experience rapidly demonstrated how these tools could streamline decision-making processes.
A powerful strategy for me is the contextual use of the tool within my 8 Gears of Success framework. I recently applied an exploratory analysis technique to identify emerging market trends, which helped refine Profit Leap's service offerings and increased our client acquisition by 15%. By embedding the learning process within ongoing business activities, I quickly grasp the tool's utility and foster continuous improvement.
Moreover, I engage in cross-disciplinary collaborations that marry healthcare insights with technology services. When expanding to São Paulo, I integrated medical imaging data into business strategies, showcasing how diverse perspectives can improve tool proficiency. This cross-industry approach allows for a richer understanding and novel applications of data tools.
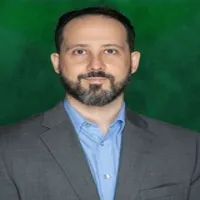
Adopt Structured Yet Flexible Approach
When our agency needed to master a new data analysis tool like Tableau, we adopted a structured yet flexible approach to ensure quick proficiency. We began by defining clear objectives, and understanding what we aimed to achieve with Tableau, such as enhancing our data visualization capabilities for client reports. To build a solid foundation, we utilized official tutorials and comprehensive documentation, allowing team members to grasp the basic functionalities and features of the tool.
One strategy that proved exceptionally effective was the "learning by doing" method. We assigned each team member a small, real-world project related to their current work, enabling them to apply Tableau's features directly to actual campaigns. This hands-on experience not only accelerated their learning curve but also demonstrated the practical benefits of the tool in our daily operations. Additionally, we fostered a collaborative learning environment by organizing regular internal workshops where team members could share their insights, discuss challenges, and showcase their progress. This collective knowledge-sharing not only reinforced individual understanding but also sparked innovative uses of Tableau across different projects.
For example, after implementing Tableau, we were able to create more detailed and interactive visualizations for our clients, leading to a 20% increase in client satisfaction scores due to the enhanced clarity and depth of our reports. My key advice is to combine practical application with continuous learning and team collaboration. By integrating real-world projects with ongoing education and fostering a supportive environment, businesses can swiftly become proficient in new data analysis tools, ultimately boosting their analytical capabilities and delivering superior results to clients.
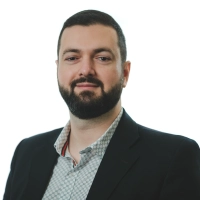
Start with a Familiar Dataset
When I need to learn a new data analysis tool or technique, I start with a small project using a dataset I already know. This helps me focus on the tool itself instead of spending time figuring out the data. A familiar dataset allows me to compare results and see how the tool handles the same problem in different ways. One time, when testing a new visualization tool, I used a security incident dataset we had previously analyzed at Parachute. This made it easy to spot differences in how the tool processed trends and flagged anomalies.
I choose a simple, relevant problem that matches my daily work but also pushes me to use the new tool's features. Learning is easier when solving a problem that matters. For example, when exploring a new log analysis tool, I used it to track failed login attempts on a network. The immediate results showed patterns that weren't obvious before. This hands-on approach not only made the tool easier to understand but also helped improve our security monitoring.
Taking notes on each step makes learning more effective. Writing down key functions, challenges, and insights helps me remember what worked and what didn't. I also experiment with different settings to see how they affect the results. Adjusting filters, testing various query structures, and trying advanced features over time builds confidence. The goal is not just to learn the tool but to understand how it can make data analysis more efficient in real-world situations.
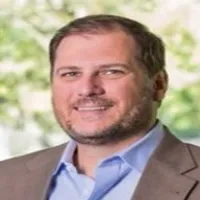
Blend Theory with Practical Application
When I dive into a new data analysis tool or technique, I prioritize blending theory with practical application. For instance, when I integrated AI analytics tools at Celestial Digital Services, I started by experimenting with real campaign data, which allowed me to uncover insights into customer behavior patterns. This hands-on approach helped me quickly understand the tool's full capabilities.
I also build prototype projects, like creating detailed SEO reports using new techniques. When exploring new machine learning tools, I crafted a project focused on predicting seasonal variations in online traffic for our clients. This method not only boosted my proficiency but also provided usable insights for our business strategies.
Staying connected within digital marketing communities is essential. Participating in forums and discussing experiences with fellow professionals has exposed me to diverse perspectives and methods that aid in efficiently mastering new tools and techniques. The collaborative dialogue accelerates learning, much like it did when we dug into data visualization techniques.
Get a Grip on Main Ideas
When I'm picking up a new data analysis tool or technique, I kick things off by getting a grip on the main ideas and the specific problems it aims to tackle. I usually start with some beginner resources, like reading up on the basics, checking out tutorial videos, or browsing online forums where people share their experiences and use cases. A key strategy that really helps me get the hang of it quickly is jumping into hands-on practice. Rather than just passively consuming information, I like to dive into a small project or dataset to put my knowledge to the test. This way, I not only reinforce what I've learned but also discover little details that might be missed in theory. It keeps me interested and boosts my confidence as I become more comfortable with what the tool can do.
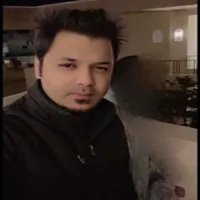
Identify Specific Use Cases
I start by identifying its specific use cases for optimizing our marketing campaigns, such as tracking customer behavior or improving ad targeting. I prioritize hands-on learning by experimenting with real campaign data, testing different features, and analyzing outputs to understand its practical applications. Online tutorials and community forums help me uncover best practices and troubleshoot challenges efficiently.
To speed up proficiency, I create a simple workflow or checklist that streamlines repetitive tasks, ensuring consistent and accurate insights. One important technique for me is to set up automated reports early on, allowing me to focus on interpreting data rather than spending excessive time on manual analysis.
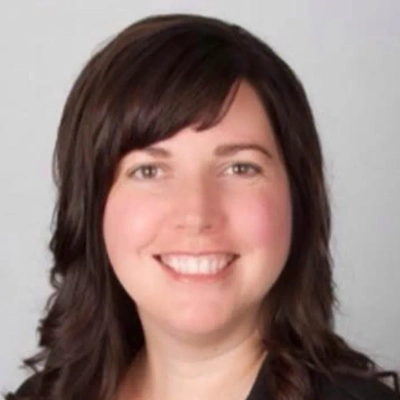
Combine Hands-On Practice with Structured Learning
When I need to learn a new data analysis tool or technique, I dive into hands-on practice combined with structured learning. For instance, when transitioning UNO DENTAL to paperless charting, I first explored the software through tutorials and then applied it to real patient scenarios. This approach allowed me to understand its practical application quickly.
A specific strategy I use is to create a mini-project or case study related to my field. For example, I examined patient retention rates, applying new data techniques to refine treatment plans. By focusing on a tangible outcome, I can grasp the tool's strengths and limitations more effectively.
Collaboration is also key. I regularly engage with my team at UNO DENTAL, sharing insights and learning from their experiences. This exchange of knowledge accelerates proficiency, as it did when we implemented intraoral scanning techniques.
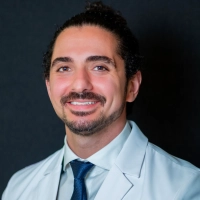