5 Strategies for Handling Outliers in Data Analysis
Outliers in datasets can make or break an analysis, and knowing how to handle them is crucial. Insights from a Data Analyst and a CEO provide the strategies you need to tackle these anomalies effectively. The first insight discusses how to identify and correct data entry errors, while the last emphasizes the importance of transforming valid outliers for accurate insights. Discover all five expert strategies to enhance your data analysis skills.
- Identify and Correct Data Entry Errors
- Isolate and Analyze Legitimate Outliers
- Understand Context and Investigate Anomalies
- Transform Valid Outliers for Accurate Insights
- Investigate Anomalies for Valuable Insights
Identify and Correct Data Entry Errors
My strategy for handling outliers involves first identifying them using statistical techniques like IQR or Z-scores and then determining whether they result from data-entry errors, system issues, or genuine anomalies. In practical analysis, I encountered a case where a query pulling activity data for a specific employee in our CRM returned null values. Investigating further, I discovered the employee's name in the CRM was misspelled compared to the 'usertable' in our MySQL database. This mismatch caused the employee's records to be excluded, acting as an outlier in the dataset. By correcting the name in the CRM to match the 'usertable', we resolved the issue and ensured accurate data reporting.
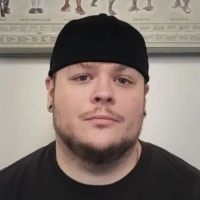
Isolate and Analyze Legitimate Outliers
In my analysis, I approach outliers by first identifying their cause—whether they stem from data entry errors or represent valid, extreme cases. For instance, while analyzing sales data, I discovered an outlier that significantly skewed the results. After investigating, I realized it was a rare but legitimate bulk purchase. By isolating this outlier for a separate analysis, I was able to draw meaningful insights without distorting the overall trends.
Understand Context and Investigate Anomalies
At Tech Advisors, we approach outliers with a clear strategy to ensure accurate data analysis and meaningful insights. The first step is understanding the context of the data and the potential impact of these anomalies. Outliers can sometimes represent errors, like a negative age in a demographic dataset, or they can highlight important trends, such as unexpected spikes in cybersecurity threats. We analyze their nature using tools like box plots, z-scores, and the interquartile range (IQR) method to identify data points that stand apart from the majority. Visualization often helps uncover patterns or discrepancies that numbers alone might miss.
Once we identify outliers, we carefully decide how to address them. During a cybersecurity audit for a client in Boston, our team found unusually high login attempts from a single IP address. Rather than removing the data outright, we flagged it for further investigation. It turned out to be an attempted security breach. This case highlighted that outliers might not always be irrelevant—they can hold critical information. In other instances, such as erroneous data entries in a financial report, we removed outliers that were clearly incorrect, ensuring the dataset remained accurate and reliable.
The key is to align outlier management with the dataset’s purpose. If the outliers are genuine but extreme values, they might require special handling, such as analyzing them separately. For example, in cybersecurity, an outlier could indicate a vulnerability that needs immediate attention. However, if they are errors, removing them avoids skewing the results. Always validate your approach with domain knowledge and consider running the analysis both with and without outliers to see the difference in results. This ensures that decisions are data-informed and contextually sound.
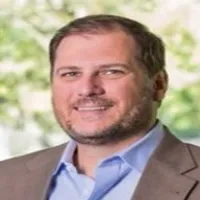
Transform Valid Outliers for Accurate Insights
At Edumentors, when analyzing student progress data, we encountered outliers in test scores that skewed the results. To handle this, we first identified the outliers using statistical methods like the IQR (Inter-Quartile Range) rule. We then analyzed if they were genuine anomalies or data entry errors. For those that were valid but extreme, we used transformations to minimize their impact on our overall analysis, which led to more accurate insights for tutoring performance. The key lesson was to balance data integrity with analytical clarity.
Investigate Anomalies for Valuable Insights
When dealing with outliers in a dataset, my strategy is to first identify the root cause of the anomaly. This involves digging deeper into the data-collection process, understanding the context in which the data was generated, and checking for any errors or inconsistencies. In one instance, I was working on a project that involved analyzing user engagement metrics for a popular social media platform. Upon reviewing the data, I noticed a significant spike in engagement rates for a particular user group.
Initially, I thought it was an outlier that could be ignored, but upon further investigation, I discovered that the spike was due to a change in the platform's algorithm that had inadvertently caused the increase. Instead of removing the outlier, I chose to explore this anomaly further, which led to a valuable insight into the platform's user behavior. This experience taught me that outliers can often provide valuable insights and should not be dismissed without proper investigation. My advice is to approach outliers with a curious mindset, and to always question the data before making any conclusions.
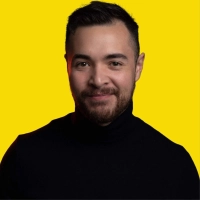