4 Common Pitfalls in Data Analysis and How to Avoid Them
Data analysis is a crucial skill in today's data-driven world, but it's not without its challenges. This article explores common pitfalls that analysts face and provides expert-backed strategies to overcome them. From understanding business context to combating confirmation bias, readers will gain valuable insights to enhance their analytical prowess.
- Understand Business Context Before Analyzing Data
- Define Problem Clearly to Guide Analysis
- Clean Data Thoroughly for Accurate Results
- Combat Confirmation Bias in Data Analysis
Understand Business Context Before Analyzing Data
One of the most common pitfalls I see data analysts fall into, especially when building dashboards for businesses, is jumping into the data without a clear understanding of the business context. When you don't fully grasp the underlying goals or decision-making needs, it's easy to answer the wrong question or track the wrong KPI. At Celsius BI, we always start with the question: what should this data enable someone to do?
Another common issue is overloading dashboards with too much information. The temptation is to show everything, but in reality, clutter kills clarity. A dashboard should guide, not confuse. One page, one clear story -- that's the principle we stick to.
Trusting the source without validating the data is another quiet pitfall. If key figures aren't double-checked, it can lead to wrong conclusions or even a loss of trust in the dashboard itself. Building in simple validation checks early in the process saves a lot of trouble later.
Many analysts also bring their own assumptions or biases into the analysis, often without realizing it. If you're only looking to confirm what you expect, you risk overlooking what the data is actually telling you. A good analyst approaches data with curiosity and a genuinely open mind -- ready to be surprised, and willing to dig deeper when things don't fit the expected pattern.
Finally, analysts often underestimate the importance of storytelling. Data without a clear narrative rarely inspires action. The most impactful dashboards are the ones that don't just show the numbers, but guide the user through what's happening, why it matters, and what to do next.
Avoiding these pitfalls doesn't just make your work more accurate -- it helps ensure your insights actually drive better decisions.
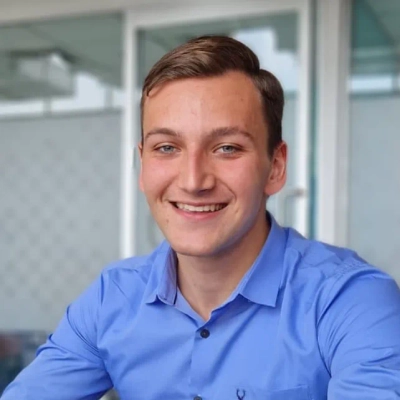
Define Problem Clearly to Guide Analysis
One of the most common pitfalls data analysts fall into is not properly defining the problem before diving into the data. If the question or objective isn't clear from the start, the analysis can end up answering the wrong thing, leading to wasted time and potentially misguided decisions. To avoid this, always take time to clarify the problem and align it with the business goal before pulling any data.
Another issue is confirmation bias—where analysts inadvertently look for data that supports a preconceived notion or hypothesis. This can skew results and lead to faulty conclusions. To avoid this, I always make it a point to explore the data objectively, using exploratory data analysis (EDA) to let the data tell its own story before jumping to conclusions.
A third pitfall is failing to account for data quality, like ignoring missing values, duplicate entries, or outliers. Poor data quality can lead to misleading results, so always perform data cleaning and validation before starting any analysis. It's tedious, but it's crucial for producing reliable insights.
The consequences of these mistakes can range from poor business decisions to lost trust in the data team. The key to avoiding them is creating clear, unbiased processes from the start, and taking the time to ensure that the data you're working with is solid and aligned with the real business questions.
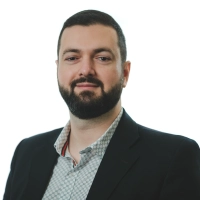
Clean Data Thoroughly for Accurate Results
When diving into the world of data analysis, it's easy to get tripped up by a few common mistakes that can significantly skew results and lead to faulty conclusions. One major pitfall is neglecting to clean and preprocess the data properly before starting the analysis. Dirty data, which can include duplicate records, inconsistencies, and missing values, often leads to misleading results and can affect the validity of the entire analysis. Another typical error is failing to understand the underlying assumptions of statistical tests or algorithms used in the analysis, which can render the results invalid if the assumptions are not met.
The consequences of these pitfalls range from minor misinterpretations to making faulty business decisions based on incorrect data. For instance, if a data analyst at a retail company overlooks the assumption checks for a linear regression model predicting sales, they might end up with results indicating that less advertising leads to more sales, completely misleading the marketing strategy. To avoid these common mistakes, analysts should ensure they dedicate sufficient time to data cleaning and validation stages. Moreover, acquiring a solid understanding of the statistical methods and tools being used is crucial. Regularly checking and double-checking each step of the data analysis pipeline can save both time and resources in the long run, besides preserving the integrity of the data-driven insights being generated. This meticulous approach helps to maintain accuracy and trustworthiness in the analysis, ultimately supporting better decision-making.
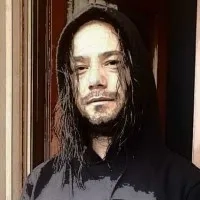
Combat Confirmation Bias in Data Analysis
One major pitfall I've encountered is confirmation bias—where analysts (myself included, early on) cherry-pick data that supports their assumptions. For example, in a marketing campaign analysis, I initially overlooked underperforming segments because they contradicted my hypothesis. This led to overestimating ROI and poor budget decisions. Now, I combat this by:
1. Defining hypotheses upfront and seeking disconfirming evidence.
2. Using blind analysis (e.g., hiding segment labels during initial review).
Another critical issue is dirty data—missing values, duplicates, or inconsistent formatting. Once, I rushed an analysis without proper cleaning, resulting in a 10% revenue overestimation. Now, I always:
1. Audit data quality before analysis (e.g., checking for outliers).
2. Document cleaning steps for reproducibility.
The consequences of these pitfalls range from misguided business decisions to loss of stakeholder trust. Rigorous processes and peer reviews are my safeguards.
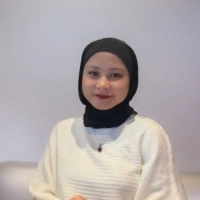